Pavel Izmailov
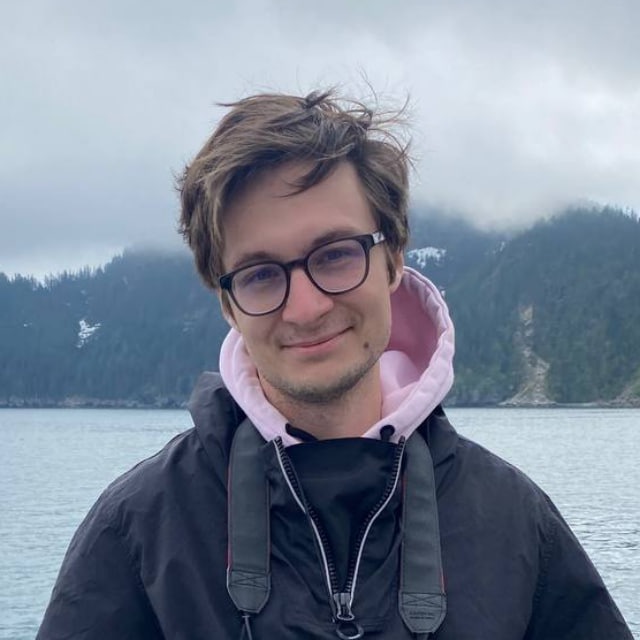
Contact: pi390@nyu.edu, Twitter
I am a Researcher at Anthropic. I am primarily interested in reinforcement learning, reasoning, AI for science and AI alignment. Previously, I worked on reasoning and superintelligent AI alignment at OpenAI.
Starting in Fall 2025, I will be joining NYU as an Assistant Professor in the Tandon CSE department, and Courant CS department by courtesy. I am also a member of the NYU CILVR Group.
My research interests are broadly in understanding how deep neural networks work. I am excited about a broad array of topics in core machine learning, including:
- • Problem-solving and reasoning in AI
- • Reinforcement learning, planning and search
- • Interpretability of deep learning models
- • AI for scientific discovery and math
- • Generalization and robustness of AI models
- • Technical AI alignment
- • Probabilistic deep learning, uncertainty estimation and Bayesian methods
Highlights
- • I contributed to the Anthropic Claude 3.7 Sonnet, a state-of-the art reasoning and coding model.
- • I contributed to OpenAI o1, a new state-of-the-art in LLM reasoning.
- • Our work on weak-to-strong generalization was covered by a WIRED, MIT Technology Review and others.
- • Our work on Bayesian model selection was recognized with an Outstanding Paper Award 🏆 at ICML 2022!
Links
- [Home, Bio, Publications, Talks, CV, GitHub, Google Scholar, Semantic Scholar]
Talks
-
Weak-to-strong generalization
University of California, Los Angeles, NLP Seminar; February, 2025
-
Debate: Sparks versus embers (discussant)
Simons Institute for the Theory of Computing, UC Berkeley; December, 2024
[video] -
Weak-to-strong generalization
Simons Institute for the Theory of Computing, UC Berkeley; December, 2024
[video] -
A Bayesian Odyssey in Uncertainty: from Theoretical Foundations to Real-World Applications
ECCV Tutorial; September, 2024
[video] -
Weak-to-strong generalization
NYU AI Safety Reading Group; May, 2024 -
Symposium on the Impact of Generative AI in the Physical Sciences (Panelist)
IAIFI, MIT; March, 2024 -
Weak-to-strong generalization
Columbia Human-Guided Machine Learning Seminar; February, 2024 -
Weak-to-strong generalization
OpenAI Forum; January, 2024 -
Neural network loss surfaces and Bayesian neural nets
Caltech, guest lecture; May, 2023 -
Feature Learning and Distribution Shift
Stanford, Chelsea Finn’s group; December, 2022 -
Understanding Knowledge Distillation
MIT, Tommi Jaakkola’s group; November, 2022 -
Feature Learning and Spurious Correlations
University of Washington, Ludwig Schmidt’s group; November, 2022 -
On Uncertainty, Tempering, and Data Augmentation in Bayesian Classification
Google Research, Shannon’s Bandwagon meeting; July, 2022 -
Last Layer Re-Training is Sufficient for Robustness to Spurious Correlations
Google Research, Sample Efficient Learning meeting; July, 2022 -
What Are Bayesian Neural Network Posteriors Really Like?
AABI Invited Talk [video] and
Max Plank Institute MIS and UCLA joint Seminar: Math Machine Learning [video] and
Teams at Google Brain and Perception and
Oxford Applied and Theoretical Machine Learning Group and
Bayesgroup seminar and
International Conference on Machine Learning (ICML);
2021 -
Does Knowledge Distillation Really Work?
Teams at Google Brain and Perception; 2021 -
Bayesian Deep Learning and a Probabilistic Perspective of Generalization
University of Freiburg, Frank Hutter's group; 2021 -
How Do We Build Neural Networks We Can Trust?
Broad Institute of MIT and Harvard MIA Seminar; Invited Talk 2019
[video] -
Scalable Bayesian inference in low-dimensional subspaces
Bayesgroup seminar; 2019 -
Subspace Inference for Bayesian Deep Learning
Harvard, Finale Doshi-Velez group; 2019 -
Averaging Weights Leads to Wider Optima and Better Generalization
UAI Oral presentation, 2018
[video] -
Scalable Gaussian Processes with Billions of Inducing Inputs via Tensor Train Decomposition
Artificial Intelligence and Statistics (AISTATS) Oral presentation; 2018