Pavel Izmailov
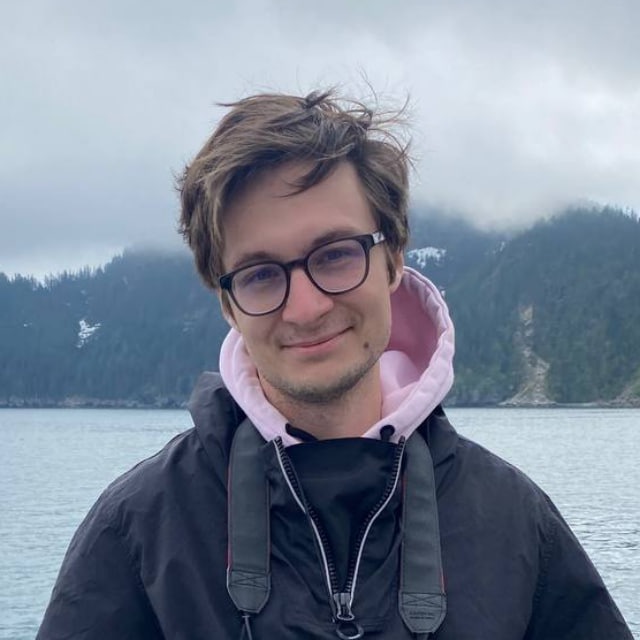
Contact: pi390@nyu.edu, Twitter
I am a Researcher at Anthropic. I am primarily interested in reinforcement learning, reasoning, AI for science and AI alignment. Previously, I worked on reasoning and superintelligent AI alignment at OpenAI.
Starting in Fall 2025, I will be joining NYU as an Assistant Professor in the Tandon CSE department, and Courant CS department by courtesy. I am also a member of the NYU CILVR Group.
My research interests are broadly in understanding how deep neural networks work. I am excited about a broad array of topics in core machine learning, including:
- • Problem-solving and reasoning in AI
- • Reinforcement learning, planning and search
- • Interpretability of deep learning models
- • AI for scientific discovery and math
- • Generalization and robustness of AI models
- • Technical AI alignment
- • Probabilistic deep learning, uncertainty estimation and Bayesian methods
Highlights
- • I contributed to the Anthropic Claude 3.7 Sonnet, a state-of-the art reasoning and coding model.
- • I contributed to OpenAI o1, a new state-of-the-art in LLM reasoning.
- • Our work on weak-to-strong generalization was covered by a WIRED, MIT Technology Review and others.
- • Our work on Bayesian model selection was recognized with an Outstanding Paper Award 🏆 at ICML 2022!
Links
- [Home, Bio, Publications, Talks, CV, GitHub, Google Scholar, Semantic Scholar]
Publications
-
*Equal first authorship.
-
OpenAI o1 System Card
2024
[arXiv] -
Learning to Reason with LLMs
2024
[OpenAI blog] -
Can a Confident Prior Replace a Cold Posterior?
arXiv preprint, 2024
[PDF, ArXiv, Code] -
Weak-to-Strong Generalization: Eliciting Strong Capabilities With Weak Supervision
2023
[PDF, ArXiv, OpenAI blog, Code] [WIRED, TechCrunch, MIT Technology Review, IEEE Spectrum] -
Simple and Fast Group Robustness by Automatic Feature Reweighting
International Conference on Machine Learning (ICML), 2023
[PDF, ArXiv, Code] -
FlexiViT: one model for all patch sizes
Conference on Computer Vision and Pattern Recognition (CVPR), 2023
[PDF, ArXiv, Code] -
Last Layer Re-Training is Sufficient for Robustness to Spurious Correlations
International Conference on Learning Representations (ICLR), 2023
🌟 Spotlight Presentation
[PDF, ArXiv, Code] -
On Feature Learning in the Presence of Spurious Correlations
Neural Information Processing Systems (NeurIPS), 2022
[PDF, ArXiv, Code] -
On Uncertainty, Tempering, and Data Augmentation in Bayesian Classification
Neural Information Processing Systems (NeurIPS), 2022
[PDF, ArXiv, Code] -
Bayesian Model Selection, the Marginal Likelihood, and Generalization
International Conference on Machine Learning (ICML), 2022
🏆 Outstanding Paper Award, 📢 Long Talk (Oral)
[PDF, ArXiv, Code] -
Unsupervised learning of two-component nematicity from STM data on magic angle bilayer graphene
arXiv preprint, 2022
[PDF, ArXiv] -
Dangers of Bayesian Model Averaging under Covariate Shift
Neural Information Processing Systems (NeurIPS), 2021
[PDF, ArXiv, Poster, Code] -
Does Knowledge Distillation Really Work?
Neural Information Processing Systems (NeurIPS), 2021
[PDF, ArXiv, Poster, Code] -
What Are Bayesian Neural Network Posteriors Really Like?
International Conference on Machine Learning (ICML), 2021
📢 Long Talk (Oral)
[PDF, ArXiv, Code, HMC samples, Poster, NeurIPS competition] -
Learning Invariances in Neural Networks from Training Data
Neural Information Processing Systems (NeurIPS), 2020
[PDF, ArXiv, Code] -
Why Normalizing Flows Fail to Detect Out-of-Distribution Data
Neural Information Processing Systems (NeurIPS), 2020
[PDF, ArXiv, Code] -
Bayesian Deep Learning and a Probabilistic Perspective of Generalization
Neural Information Processing Systems (NeurIPS), 2020
[PDF, ArXiv, Code] -
Generalizing Convolutional Neural Networks for Equivariance to Lie Groups on Arbitrary Continuous Data
International Conference on Machine Learning (ICML), 2020
[PDF, ArXiv, Code] -
Semi-Supervised Learning with Normalizing Flows
International Conference on Machine Learning (ICML), 2020
[PDF, ArXiv, Code] -
Subspace Inference for Bayesian Deep Learning
Uncertainty in Artificial Intelligence (UAI), 2019
[PDF, ArXiv, Code, Poster] -
A Simple Baseline for Bayesian Uncertainty in Deep Learning
Neural Information Processing Systems (NeurIPS), 2019
[PDF, ArXiv, Code, Poster, Video] -
There Are Many Consistent Explanations of Unlabeled Data: Why You Should Average
International Conference on Learning Representations (ICLR), 2019
[PDF, ArXiv, Code, Poster] -
Averaging Weights Leads to Wider Optima and Better Generalization
Uncertainty in Artificial Intelligence (UAI), 2018
📢 Oral Presentation
[PDF, ArXiv, Code, Poster, Slides, PyTorch Blogpost, Towards Data Science Blogpost, fast.ai Blogpost] -
Loss Surfaces, Mode Connectivity, and Fast Ensembling of DNNs
Neural Information Processing Systems (NeurIPS), 2018
🌟 Spotlight Presentation
[PDF, ArXiv, Code, Poster, Slides, Video, Blogpost] -
Tensor Train decomposition on TensorFlow (T3F)
Journal of Machine Learning Research, 2020
[PDF, ArXiv, Code] -
Scalable Gaussian Processes with Billions of Inducing Inputs via Tensor Train Decomposition
Artificial Intelligence and Statistics (AISTATS), 2018
📢 Oral Presentation
[PDF, ArXiv, Code, Poster, Slides] -
Faster variational inducing input Gaussian process classification
Journal of Machine Learning and Data Analysis, 2017
[PDF, ArXiv]
Workshop Papers
-
On Feature Learning in the Presence of Spurious Correlations
ICML Workshop on Principles of Distribution Shift (PODS), 2022
-
Last Layer Re-Training is Sufficient for Robustness to Spurious Correlations
ICML Workshop on Spurious Correlations, Invariance, and Stability, 2022
📢 Oral Presentation
[PDF, ArXiv, Code] -
Semi-Supervised Learning with Normalizing Flows
ICML Workshop on Invertible Neural Nets and Normalizing Flows, 2019
[PDF, Poster] -
Invertible Convolutional Networks
ICML Workshop on Invertible Neural Nets and Normalizing Flows, 2019
🌟 Spotlight Presentation
[PDF, Poster, Slides] -
Subspace Inference for Bayesian Deep Learning
ICML Workshop on Uncertainty & Robustness in Deep Learning, 2019
📢 Oral Presentation
[PDF, ArXiv, Code, Poster, Slides, Polina's Talk] -
Fast Uncertainty Estimates and Bayesian Model Averaging of DNNs
UAI Workshop: Uncertainty in Deep Learning, 2018
📢 Oral Presentation
[PDF, Code, Poster, Slides] -
Improving Stability in Deep Reinforcement Learning with Weight Averaging
UAI Workshop: Uncertainty in Deep Learning, 2018
[PDF, Poster]